It seems no matter where you look online now, there is an article about Artificial Intelligence (AI), specifically Generative AI and how it is / has / will change the world. However, one thing I have noticed is that there is a lot of confusion about the subsets, especially Machine Learning (ML) and excluding GPT in the main a lot of what we are seeing is ML and not AI.
I thought I would write something to help explain the difference across the interesting world of AI as simply as possible, followed with a handy three key differences.
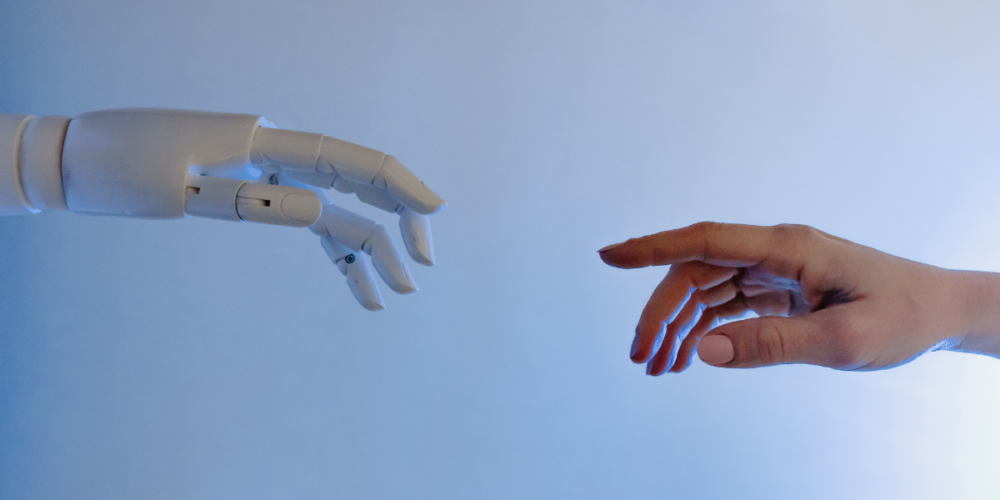
At its most basic Artificial Intelligence (AI) is a broad field that encompasses several subfields.
- Machine Learning (ML): Focuses on the development of algorithms that enable machines to learn from data without being explicitly programmed. ML algorithms use data to identify patterns and make predictions or decisions.
- Natural Language Processing (NLP): NLP is the interaction between machines and human language. It involves developing algorithms that enable machines to understand, interpret, and generate human language.
- Speech Recognition (SR): Not to be confused with NLP, SR focuses on enabling machines to recognize and interpret human speech. Speech recognition algorithms can be used for applications such as voice assistants, speech-to-text software, and phone-based customer service systems.
- Computer Vision: Focuses on enabling machines to understand and interpret visual information from the world around them. Computer vision algorithms can analyse images and video to identify objects, people, and other visual features.
- Robotics: Robotics is the development of machines that can perform physical tasks. Robots can be programmed to perform a variety of tasks, from simple assembly line operations to complex surgeries.
- Expert Systems: Are designed to emulate the decision-making capabilities of a human expert in a specific domain. These systems use rules and data to provide advice or make decisions based on the input they receive.
- Cognitive Computing: Developing systems that can mimic human thought processes. These systems use machine learning, NLP, and other techniques to analyse and interpret complex data sets. Therefore, much closer to what we call ‘AI’
These subfields of AI are often interconnected and can be used together to develop more advanced systems. As AI research continues to evolve, we may see the emergence of new subfields and applications.
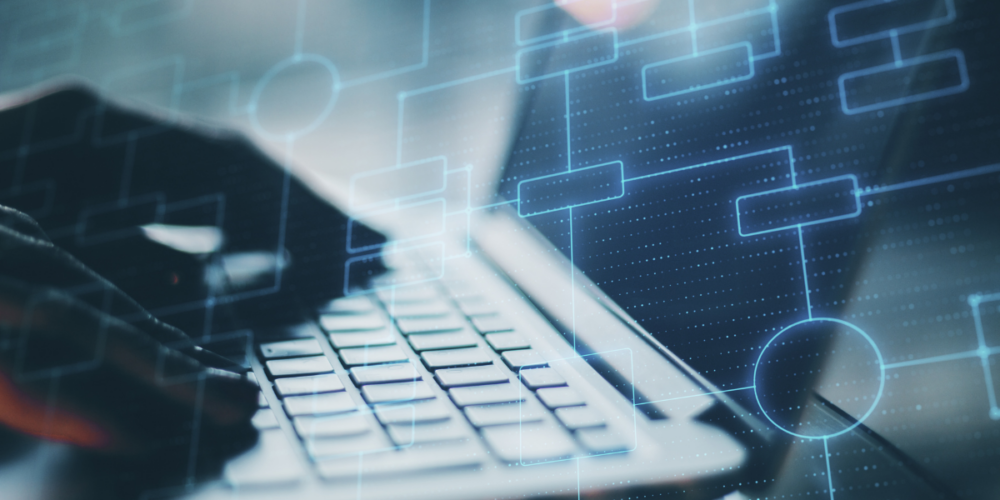
ML vs AI
The common misconception is that Machine Learning (ML) is equivalent to Artificial Intelligence (AI). However, as you can see from above it is a subset of AI and there are several reasons why the assumption is untrue:
- ML is a subset of AI that focuses on developing algorithms that enable machines to learn from data and make predictions or decisions. ML algorithms work by processing large amounts of data and identifying patterns, allowing the machine to improve its performance over time. However, ML algorithms are still based on predefined rules and require human intervention to set parameters and evaluate results. In other words, ML systems can perform specific tasks but lack the ability to reason or understand the context in which those tasks are performed.
- True AI is a broader concept that encompasses not only machine learning but also other subfields, such as natural language processing, computer vision, robotics, and expert systems. True AI aims to create machines that can perform tasks that require human intelligence, such as perception, reasoning, learning, and problem-solving. These machines can understand the context in which they operate and adapt to new situations without human intervention.
- ML algorithms are based on statistical models that are trained on historical data. These algorithms can be biased if the training data is not representative of the real-world context in which the machine operates. For example, an ML system that is trained on data from a specific demographic may not perform well when deployed in a different demographic. On the other hand, true AI systems can adapt to new situations and learn from experience, without being limited by biased training data.
While Machine Learning is a powerful tool that has enabled significant progress in many areas, it is not true AI. ML algorithms are still based on predefined rules and require human intervention to set parameters and evaluate results. True AI, on the other hand, aims to create machines that can reason, understand, and learn from experience without human intervention. Overall, AI is a rapidly growing field with the potential to revolutionize many aspects of our lives. As technology advances, we can expect to see more sophisticated AI systems that are capable of performing increasingly complex tasks.
Hopefully this article proves helpful in understanding what everyone is talking about or if you are in the process of being miss sold AI!